The Physics of Noise
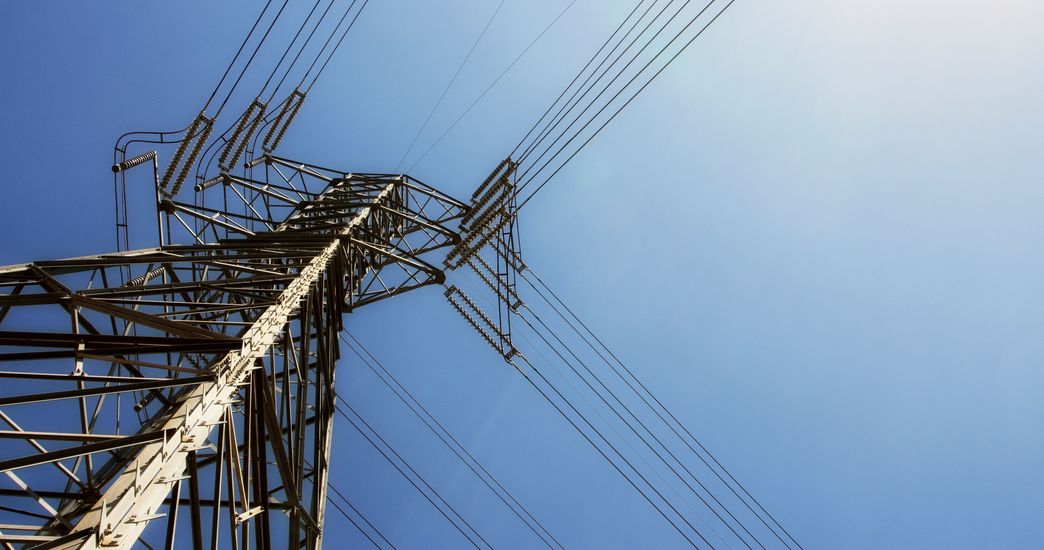
HDS-LEE alumnus Leonardo Rydin Gorjão has been researching how we can ensure a constant power supply if we only get it from renewable energy sources - using data science methods. HIDA's exchange programs have enabled him to spend time at various Helmholtz centers and to network closely within the community.
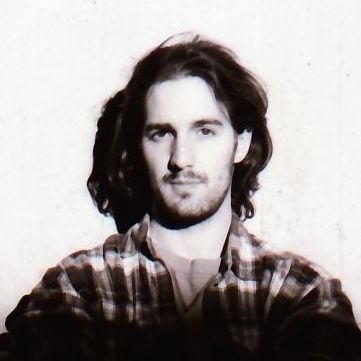
Our interview partner Leonardo Rydin Gorjão
Our interview partner Leonardo Rydin Gorjão
The Portuguese physicist Leonardo Rydin Gorjão, 34, – now associate professor at the Norwegian University of Life Sciences (NMBU) in Ås – was a doctoral candidate at the Helmholtz School for Data Science in Life, Earth and Energy (HDS-LEE) in Jülich. He took part in the HIDA Trainee Network and the Norway Exchange program.
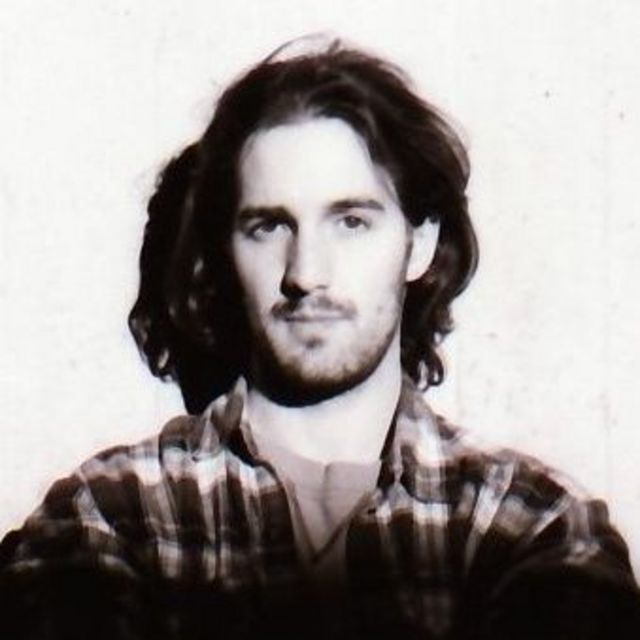
For your dissertation you chose a topic from the field of renewable energy. The title of your thesis was: “Stochastic time series analysis in electric power systems and paleo climate data”. Can you describe what you were researching on?
Let’s start by saying that it is a topic that appears a lot in the news nowadays. My research was centered around the question: How can we reliably maintain power delivery in power grids? Can we constantly deliver power if, for example, we are only obtaining it from the sun or from the wind? These power sources are usually called “volatile” or “intermittent”. Some people would say: unreliable. That is one of the questions I wanted to research – is it really unreliable? Mathematicians describe these processes with stochastic processes, which in themself comprise a large research field in mathematics. This field can be boiled down to the mathematics of data and chance, and the study of noise, as we call it.
Why were stochastic processes interesting for your research questions?
They give us one way to analyze and simulate the “volatile” nature of power systems, which can negatively impact the stability of a power grid system. There are various time scales at which potential problems emerge. For example, renewable wind power generation varies rapidly in a period of a few seconds, swaying up and down, but it similarly increases and decreases from night to day. Moreover, it varies in intensity over the range of a week, sometimes vanishing completely for as long as seven straight days. My research focused on measurable effects from power generation seen at the level of power-grid frequency – which we commonly remember as 50 Hertz.
The impact of these fluctuations is detrimental to power systems equipment and the generators themselves. This always results in the question whether renewable energies are reliable. I have no concern in stating that they absolutely are – we know about the consequence of their use in power systems and know how to tackle it. Research only helps us improve our knowledge and find better solutions to increase our use of renewable sources.
To understand the complexity of energy systems is something the world needs now. It is a problem we need to solve.
Leonardo Rydin Gorjão
How did you approach your research?
So for my thesis I focused on the data: What kind of data do we have? What does the data look like? What properties does it show? Can we look at the data of all kinds of energy sources and answer the question: Is it really volatile? Is there a large amplitude of fluctuation or noise in the data? That was the largest part of the thesis. The second part revolved around paleo-climate data – that is the climate of the past, and some techniques to analyze past processes and their noise.
I should note that these topics are very disconnected, yet, as a physicist, I wanted to be able to understand different processes using a similar mathematical framework. Moreover, studying the climate of the past – some of it dating back as far as 100,000 years ago – is important to understand the climate of the present.
How come you chose this topic? Did you select it because it is a current topic?
What drove me in the first place was actually not the topic itself, but the models. I was keen to understand the physics behind the noise which can to some extent have very similar properties – be it in the data from energy systems, climate records or stock markets. I wanted to understand the nature of those processes. But then I said to myself: If I am searching for tools to understand a problem, I should choose a topic that makes sense solving. And to understand the complexity of energy systems is something the world needs now. It is a problem we need to solve.
From 2019 until 2021 you were part of the first cohort of doctoral researchers at the Helmholtz School for Data Science in Life, Earth and Energy (HDS-LEE) in Jülich. Why did you apply for this data science school?
It was actually a fairly easy decision: After completing my master's degree in Physics at the University of Bonn in 2018, I joined the Forschungszentrum Jülich to start my doctoral thesis. I had already been a doctoral candidate for almost a year when I heard about the HDS-LEE. The graduate program was new at the time and it focused primarily on data science – just like my work. So I thought: perfect!
Can you describe your interest in data science?
I was always curious about what we can learn from data – more than what we can learn from the perspective of theorizing. Classical physics tended to go this way: You made a hypothesis about something, then you went out into the field, tested it for years and came back with the answer – yes or no. But if you ask modern day scientists, this is in most cases no longer how to treat the problem. To really understand how things are, you have to start with the data. The data are going to tell you the answer before you necessarily have a theory about it. This is, for example, very obvious for climate scientists.
The whole climate process is far too complex for someone affirm: I have a theory about it! Instead, you collect as many pieces of data as you can and try to put them together into something coherent. The interesting process really starts there: You have the data – but what do you actually see? And why? What can you learn from it? And how do you get from the data to a theory that you can present?
The HDS-LEE is one of six data science research schools of the Helmholtz Association. What was it like to be there?
I was really happy to be part of such a network. But I have to admit: When I joined the HDS-LEE, I had no idea how big the Helmholtz Association really is. Then I started counting the research centers – and saw the world opening up!
There are 18 research centers within the Association in all parts of Germany...
And there was a lot of involvement between the doctoral researchers of all six Helmholtz’ data science schools. They made us interact a lot. And as I did my PhD in the middle of the pandemic, we gathered a lot of online meetings and presented each other our work.
Did you make good connections this way?
Definitely! During one of those presentations, I and another doctoral candidate from HDS-LEE realized we were asking very similar questions regarding data, even though his research project was completely different from mine. His work was centered on machine learning models – “black boxes” – in particular: generative adversarial networks. These models are used for the prediction of very complicated processes: One basically has a large collection of data and wants to predict possible futures scenarios from it. That’s why you construct a machine learning model with the data in order to find answers out of it.
Research-wise I was coming from the other side – asking: What can we learn from the data that is coming out of your magic machine? Is the physics behind the new data still the same? Or have these “black boxes” somewhat altered the nature of the data? We were both curious about that. I had some methods to investigate it, which I frequently used in my research projects. We started talking and working together. This was one of the unexpected results of joining HDS-LEE – to immediately find a connection in a gathering of doctoral researchers!
This exchange brought me something that is – in my view – very important at the end of any PhD. I started looking beyond my own research topic at the bigger world out there.
Leonardo Rydin Gorjão
During your time at HDS-LEE, you participated in the HIDA Trainee Network – an interdisciplinary exchange program within the Helmholtz Association for data scientists. Where did you go?
The Trainee Network took me to the Deutsches Zentrum für Luft- und Raumfahrt (DLR) in Oldenburg. I was curious to know more about how to build computational models that one can use to simulate what happens in power grids. In this field they have much more expertise than I have. With the HIDA Trainee Network I had the chance to join them in online meetings – as it all happened during the pandemic. I learned a great extent about their methods, skills and instruments and the kind of questions they are dealing with. Various engineers at the DLR are working within the smallest time-scales in power grid systems: They are simulating what happens in power grids within microseconds and try to find out whether their machines are misbehaving or being negatively affected by renewable energy sources.
Would you recommend the Trainee Network as a research option?
Without a doubt! To be able to join another Helmholtz institute for two months was one of the best opportunities I could have imagined. I hope that the doctoral and postdoctoral researchers at Helmholtz make use of these opportunities even more. Helmholtz is one of those institutions in Germany where you will undoubtedly find someone who is doing similar research to you. It might not be the person sitting next door, it might be someone sitting on the other side of Germany. But they are there – and now you have an opportunity to collaborate with and visit them!
Did the Trainee Network help you in your career planning?
This exchange brought me something that is – in my view – very important at the end of any PhD. I started looking beyond my own research topic at the bigger world out there. What are my colleagues doing? What are the bigger research questions in my field? And what are my colleagues throughout Germany working on? What challenges did they face and how did they overcome these?
Our international exchange programs
Our international exchange programs
We promote data science collaborations across national borders with our international exchange programs:
After your dissertation you worked with scientists from Norway and had a scholarship in Japan. Is research abroad important for young scientists?
It depends a lot on the topic you are studying. For a long time, it was the standard within academia to study somewhere and then go abroad to see how things work elsewhere. Nowadays with all the possibilities of online collaboration you don’t necessarily have to move elsewhere in order to do very competent research. But it is very important to know that other research mentalities exist in different parts of the world. If you, for example, only work in German institutions, you will only be asking “German-centric” research questions – and that is what changes if you collaborate internationally. It broadens your view. To me that is the key point.
Today you are based at the Norwegian University of Life Sciences (NMBU) in Ås, about 30 kilometers south of Oslo. From there you returned to Helmholtz for a research visit with HIDAs Norway Exchange Program. What were your experiences?
This exchange program is a collaboration between the Norwegian Artificial Intelligence Research Consortium (NORA) and its partner institution HIDA. As a PhD or postdoc, you can apply for accommodation and travel grants around a self-organized research project. This gave me the chance to visit Benjamin Schäfer and his research group at the Karlsruher Institut für Technologie (KIT) in December 2022 who similarly works with machine learning applications to power-grid systems. It allowed me to attend a major conference in South Africa and strengthen the collaboration with the Grid Related Research Group, led by Jacques Maritz, at the University of the Free State.
Moreover, as I started my new appointment as associate professor in physics at NMBU in January 2023 and still had budget left, I invited a promising student from the Forschungszentrum Jülich to visit NMBU. For three weeks we worked together here in Ås on machine learning applications for electricity price forecasting. I certainly hope to see more people taking advantage of all the collaboration initiatives from Helmholtz and their research network.
The interview was conducted by Andrea Walter